Technology Vision 2023 | When Atoms meet Bits #TechVision Foundation model workings 185 There are two key innovations making this new ways. Generally speaking, more parameters lets wave of AI possible. The 昀椀rst is transformer models, a model soak up more information from its training 183 introduced by Google researchers in 2017. One of data and make more accurate predictions later. But One of the newest the newest classes of AI models, transformers are what OpenAI demonstrated with GPT-3 is that vastly classes of AI models, neural networks that identify and track relationships increasing the number of parameters in a transformer in sequential data (like the words in a sentence), model, and the computational power put into training transformers are neural to learn how they depend on and in昀氀uence each it, leads not just to higher accuracy but also the ability networks that identi¡ other. They are typically trained via self-supervised to learn tasks the model was never trained on. and track relationships learning, which for a large language model could in sequential data mean pouring through billions of blocks of text, This novel learning ability—also known as few-shot hiding words from itself, guessing what they are and zero-shot learning—means that foundation (like the words in a based on surrounding context, and repeating until it models can successfully complete new tasks given sentence), to learn how 184 can predict those words with high accuracy. This only a few or no task-speci昀椀c training examples. they depend on and technique works well for other types of sequential DeepMind’s Flamingo—an 80B parameter multimodal data too: some multimodal text-to-image generators 186 in–uence each other. visual-language model—is especially good at this. work by predicting clusters of pixels based on their In a 2022 paper, DeepMind researchers demonstrated surroundings. how Flamingo can conduct few-shot learning on a wide range of vision and language tasks, only being prompted by a few input/output examples and The second innovation is scale—signi昀椀cantly increasing the size of models, and subsequently, the without the researchers needing to change or adapt amount of compute used to train them. The size of the model’s weights. In six of 16 tasks they tested, a model is measured in parameters, which are the Flamingo surpassed state-of-the-art models that values or weights in a neural network that are trained had been trained on much more task-speci昀椀c data, to respond to various inputs or tasks in certain despite not having any re-training itself.
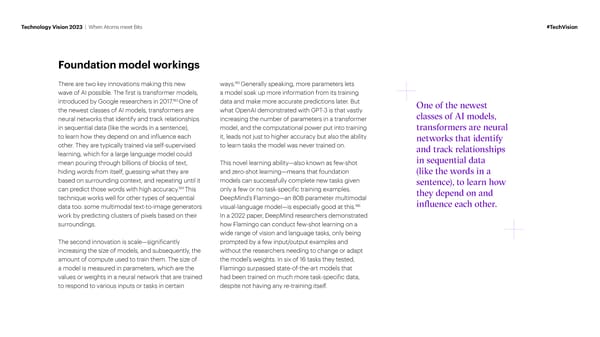