Technology Vision 2023 | When Atoms meet Bits #T#TechVechVisionision The second major bene昀椀t is that foundation models are opening the door to new AI applications and services that were dif昀椀cult or impossible to build before. For instance, lack of training data is a major issue for most Sidebar: Synthetic data and foundation models organizations looking to expand their use of AI. But with zero- and few-shot learning abilities, pretrained The intersection of data and foundation foundation models may help circumvent this limitation. models presents an interesting irony. These models require huge amounts of data for Foundation models require massive amounts of training, but once trained, can generate data upfront, which is handled by their creators. But limitless quantities of synthetic data. once a model is trained, organizations can adapt On the 昀氀ip side, as companies develop new it to a range of downstream tasks, building new This could be useful for many organizations foundation models, they may also run out constrained by the lack of real-world capabilities with just a few examples or 昀椀ne-tuning a of training data. Most language and image model with just a small training set. Rather than every labeled data, the need for data from models rely on huge amounts of web data, new AI application requiring months of effort and edge cases, or the need to preserve data and organizations’ ability to increase model investment, organizations will be able to create and privacy. Synthetic data is primed to play size depends on 昀椀nding new data sources. deploy them much more simply. IBM, for instance, has an important role in training and validating Additionally, developing foundation models been transitioning some of its Watson portfolio to use autonomous vehicles, systems that work for new modalities may require huge 205 They found that with pretrained foundation models. with health records or 昀椀nancial data, and amounts of hard-to-obtain data. Synthetic language models, Watson NLP could train sentiment much more. data, perhaps even from early foundation analysis on a new language with only a few thousand models, could be a powerful tool for sentences, a training set a hundred times smaller than building these new models. what previous models required. Over about a year, the company was able to expand Watson NLP from 12 languages to 25.
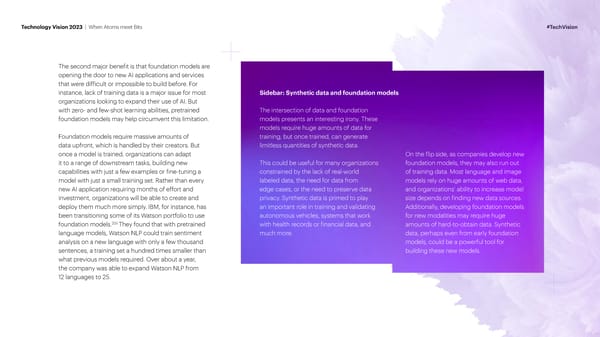